Discovering the Power Couple - How AI and Big Data Empower Each Other
There were 5 exabytes of information created between the dawn of civilization through 2003, but that much information is now created every two days. -Eric Schmidt, Executive Chairman at Google
Artificial Intelligence (AI) and Big Data are two buzzwords that have become almost inseparable in our increasingly digital world. They are two sides of the same coin, fueling the other's capabilities and creating a symbiotic relationship reshaping industries. Herein, I will delve into this fascinating relationship and try to examine how AI and Big Data form synergistic partnerships.
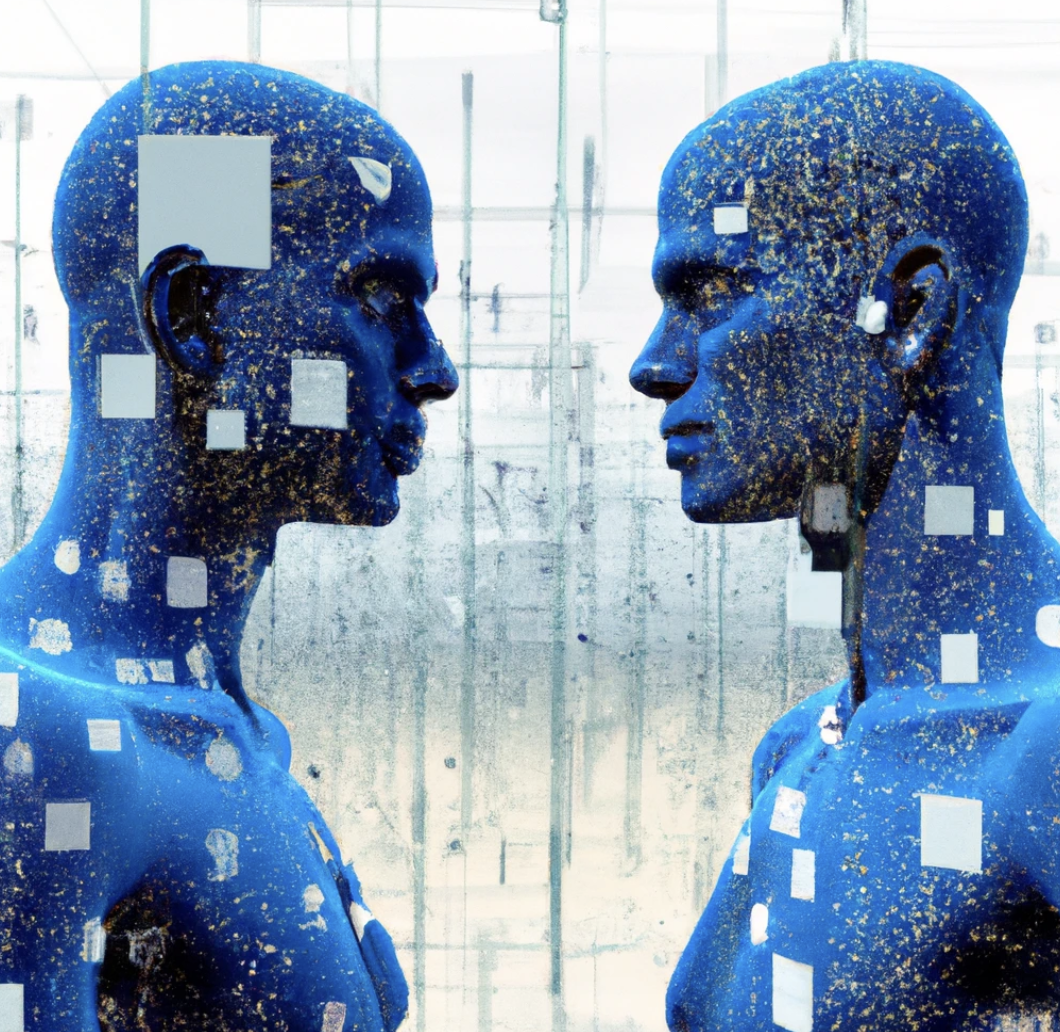
Big Data refers to massive volumes of data that are too complex for traditional data processing software. This data comes from various sources, including social media feeds, IoT devices, and business transactions, exhibiting the five Vs.: Volume, Velocity, Variety, Veracity, and Value. For example, Facebook users upload 147,000 photos every minute, and Twitter users send 500,000 tweets. This constant stream of data is a goldmine of insights, but it is also a challenge to store, process, and analyze due to its sheer volume, speed, and variety. Conversely, AI is a broad field of computer science that simulates human intelligence processes by machines, especially computer systems. AI can take several forms, such as Machine Learning (ML), where systems learn from data, identify patterns, and make decisions, and Deep Learning, a subset of ML that mimics the human brain's functioning to process data and create patterns for decision making.
AI applications are vast and range from voice assistants like Siri and Alexa to autonomous vehicles, disease diagnosis in healthcare, and personalized recommendations on streaming platforms.
AI and Big Data intersect at several points, and one of the most crucial aspects is that AI thrives on data. The more high-quality data an AI model can access, the better it can learn and make accurate predictions. For instance, recommendation systems on platforms like Amazon and Netflix are powered by ML algorithms trained on vast user data. They analyze user behavior, preferences, and buying patterns to suggest products or movies users might like. The more data these algorithms have, the better their recommendations become, enhancing user experience and boosting platform engagement. While Big Data provides the fuel for AI, AI, in return, brings sophistication to Big Data analytics. Traditional data processing methods need help with the volume, variety, and velocity of Big Data. However, with its advanced ML algorithms, AI can efficiently process and interpret this data, uncovering hidden patterns and actionable insights. AI-powered data analytics can help businesses understand customer behavior, optimize operations, predict trends, and make data-driven decisions. For instance, AI can analyze social media sentiment to gauge brand perception or predict factory machine failure by analyzing sensor data, enabling proactive maintenance.
The relationship between AI and Big Data is synergistic – they mutually enhance each other's capabilities. Big Data provides the rich, diverse datasets needed for training robust AI models, and AI brings the computational intelligence necessary to decipher Big Data's complexity. This synergy offers enormous potential. AI and Big Data can drive personalized customer experiences, enable predictive analytics, optimize business operations, and even fuel healthcare and climate science breakthroughs. They jointly power the Fourth Industrial Revolution, transforming businesses and shaping our future. However, the union of AI and Big Data is challenging. Data privacy is a significant concern, as businesses must ensure they collect and use data responsibly, adhering to regulations like GDPR. There is also the challenge of data quality - AI models trained on partial or incorrect data can produce poor results, a case of 'garbage in, garbage out.' Ensuring the data-feeding AI systems are accurate, unbiased, and representative is crucial. Moreover, the potential for AI and Big Data misuse is a serious concern. From deep fakes that can spread misinformation to intrusive surveillance systems, the ethical implications of these technologies are profound and necessitate careful regulation and oversight.
The relationship between AI and Big Data is powerful and together, they drive unprecedented technological, business, and societal advances. However, navigating this landscape with a keen awareness of the challenges and ethical implications is equally essential. Ensuring data privacy, maintaining data quality, and fostering ethical, responsible use of AI and Big Data are paramount as we harness these technologies to shape a better future.
In conclusion, the journey of AI and Big Data is far from over. As they continue to evolve and intertwine, one thing is sure: they are set to redefine our world in ways we are just beginning to imagine. This is the exciting, transformative potential of the synergistic partnership between AI and Big Data. Let us embrace it responsibly and look forward to the possibilities it holds.
Hello, I work for CodeIT, a…
Hello, I work for CodeIT, a software development company that specializes in web and mobile solutions. I enjoyed reading your topic and I agree that AI and big data are a powerful combination that can create value and solve problems. I have some experience with using AI and big data for different domains, such as healthcare, education, and e-commerce. One of the projects that I’m proud of is using AI and big data for stock prediction. You can read more about it here: Machine Learning for Stock Prediction | CodeIT. I would love to hear your opinion and suggestions on our work. Thank you!