The Doctor Will See You Now: How AI is Empowering Public Health Decision-Making
The COVID-19 pandemic has had a profound impact on public health, with millions of people worldwide affected by the virus. In response, governments, healthcare providers, and researchers have been using a range of tools and technologies that were built on innovation that was often refocused from the original objectives or assembled from existing tools and libraries, to provide insight on the evolution of the pandemic, manage the spread of the virus and improve public health outcomes. Either we believe it was a one time to forget or the beginning of a new health security era, this period has undoubtedly disrupted the otherwise rather conservative healthcare sector, where it took much effort to implement novel methods either for capturing insight from the data collected or to visualise that data. I took part of startup offering novel data preprocessing and visualisation methods (allowing for AI capabilities for, e.g., identification of biomarkers) aligned with the classical procedures in the analysis of genomic data, and have seen in first hand how difficult it is the innovation adoption here. The pandemics not only accelerated the digitalisation of the health sector but also improved the evaluation and selection of digital tools including artificial intelligence (AI), which has been increasingly used worldwide much improving classical methods. A good example is that of the excellent work that my colleague Dejan Štepec and his team is doing in AI-automated cancer cell segmentation and healthy cell generation for computer vision algorithm learning.
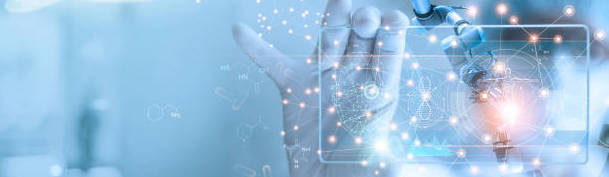
A range of digital technologies were deployed to analyse large amounts of data in real-time, providing insights and predictions that would be difficult or impossible to obtain using traditional methods. For example, AI algorithms can be used to analyze patient data to identify individuals who are at higher risk of developing severe COVID-19 symptoms, or to identify potential drugs that can be used to treat COVID-19. One of the most significant applications of AI in the fight against COVID-19 has been in the development of vaccines, where AI can be used to analyze the genetic makeup of the virus and predict which parts of the virus are most likely to elicit an immune response (information that can be used to develop more effective vaccines). Another way in which AI was used is to analyze data from social media and other sources to monitor the spread of the virus and predict where outbreaks are likely to occur, as well as in the development of contact tracing apps for managing the spread of the virus, which has shown challenges in the implementation on a large scale. Contact tracing apps used machine learning algorithms to identify individuals who may have been exposed to the virus based on their proximity to individuals who have tested positive for COVID-19. AI was also used to improve the accuracy and speed of COVID-19 testing, analyzing images of test samples and identifying whether the sample contains the virus, detecting the virus more quickly and accurately than traditional methods, which can be time-consuming and prone to errors.
When COVID-19 struck Europe in early 2020, we were releasing the MIDAS platform, developed with the European Commission for integrating big data analytics and AI-based services supporting public health policy-making. It has proven its value, applicability, and adaptability during the swift spread of the COVID-19 outbreak, already addressing priorities related to the pandemic (e.g. childhood obesity and diabetes type 2, or mental health), and allowing to include other topics present in the healthcare data ingested. By connecting various isolated and diverse data sources, including open and social data, the MIDAS platform can generate comprehensive datasets that can be analyzed, monitored, and researched. Public health authorities in Spain, Ireland and the UK utilised MIDAS to gain a better understanding of health conditions and their impact in society, track the pandemic's development across different demographics, promote increased resilience against the global emergency's repercussions, and improve preparedness for future public health crises. This happened in parallel to IRCAI's release of the Coronaviruswatch engine fed by open data and providing comparative views across different perspectives, to which we added the MEDLINE mining capabilities developed within MIDAS. Later on I published a comprehensive article about this contribution to the global COVID-19 strategy at the IEEE Computational Intelligence Magazine. In addition, MIDAS has integrated and defined a governance and ethical review model that addresses the complex privacy and ethical issues that have emerged as a result of the ongoing pandemic. This ensures that users of the system can access richer and more extensive data sources while being monitored and overseen.
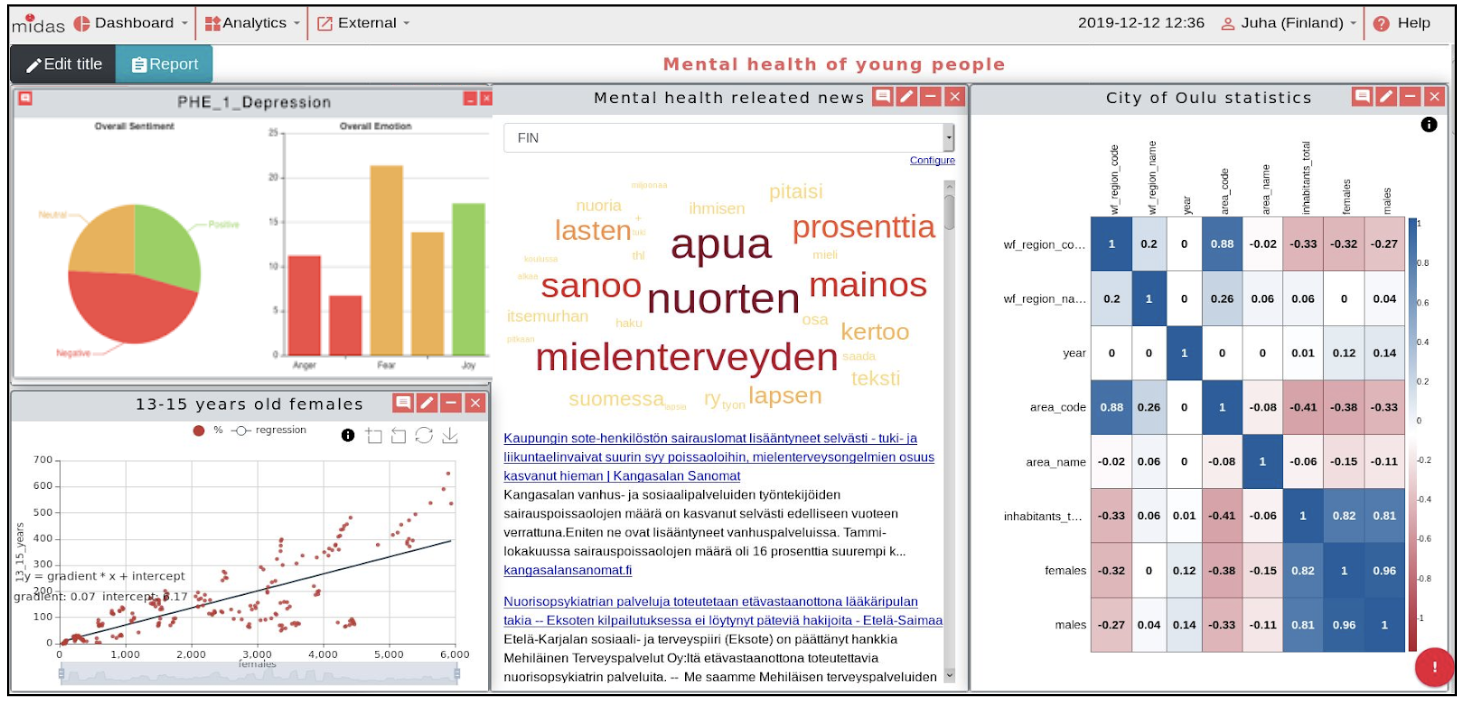
At the time I was leading research using state of the art machine learning capabilities focusing on the use of text mining tools developed to support public health institutions to complement their data with other accessible open data sources, optimise analysis and gain insight when examining policy. In particular, we focused on the exploration of MEDLINE, the biggest structured open dataset of biomedical knowledge, underneath the well known biomedical search engine Pubmed. MEDLINE has a rich terminology for indexing and cataloguing biomedical information – the MeSH headings – that can maximise the efficacy of the healthcare data ingested. Straightforward examples that we built on MEDLINE are: (i) a literature review tool allowing to use powerful queries based on Lucene language, clustering the main subtopics related to the search topic; (ii) a MeSH classifier that can assign the main medical categories in MEDLINE to any text report in english language; and (iii) a public health search engine NewsMeSH based on a variation the Eventregistry.org (that we will further discuss in one of our following posts), and built on the classification of news based on those medical categories (some code is available open source on Github). Back in 2005, while doing my pure mathematics studies, I integrated a multidisciplinary team in the Gulbenkian Science Foundation aiming for the crowdsourcing of efforts to manage a pandemics that blocks the healthcare services: Influenzanet. At the time we were focusing on the common flu, discussing a far from sight scenario that I never really imagined I would ever live it in full (and contribute to the global fight against it).
Hopefully we won’t go through an anxious time as that of COVID-19 so soon, but even if we do we know that were are more ready than we ever were with the potential shown by AI in transforming healthcare, improving diagnosis accuracy, speeding up drug development, and personalising treatment plans. While there are challenges to be addressed, such as privacy concerns and the need for more extensive data sets, the benefits of AI in healthcare are significant. With continued research and development, AI can make healthcare more efficient, effective, and accessible for patients around the world. Do you feel ready for the health challenges of tomorrow? Share your thoughts (and experiences)!